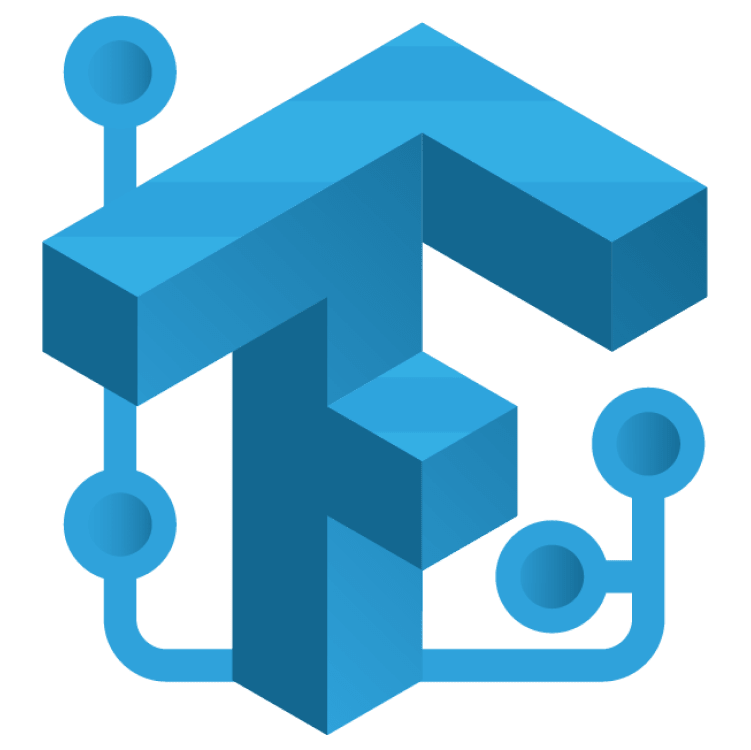
TensorFlow Lite Tutorial for Flutter: Image Classification
Learn how to use TensorFlow Lite in Flutter. Train your machine learning model with Teachable Machine and integrate the result into your Flutter mobile app. By Ken Lee.
Sign up/Sign in
With a free Kodeco account you can download source code, track your progress, bookmark, personalise your learner profile and more!
Create accountAlready a member of Kodeco? Sign in
Sign up/Sign in
With a free Kodeco account you can download source code, track your progress, bookmark, personalise your learner profile and more!
Create accountAlready a member of Kodeco? Sign in
Contents
TensorFlow Lite Tutorial for Flutter: Image Classification
30 mins
- Getting Started
- Brief Introduction to Machine Learning
- What is Machine Learning
- Training a Model: How it Works
- Understanding Tensor and TensorFlow Prediction
- Installing TensorFlow Lite in Flutter
- Creating an Image Classifier
- Importing the Model to Flutter
- Loading Classification Labels
- Importing TensorFlow Lite Model
- Implementing TensorFlow Prediction
- Pre-Processing Image Data
- Running the Prediction
- Post-Processing the Output Result
- Using the Classifier
- Picking an Image From the Device
- Initializing the Classifier
- Analyzing Images Using the Classifier
- Where to Go from Here?
Training a Model: How it Works
TensorFlow uses an approach called deep learning, which is a subset of machine learning. Deep learning utilizes a network structure with many layers, similar to what’s shown below:
To explain it further:
- The input data feeds into the first layer: If the input data is an image, the pixels of the image feed into the first layer.
- The output result is stored in the last layer: If the network is solving a classification problem, this layer stores the possibility of each class.
- The layers in between are called hidden layers. They contain formulas with parameters that sit in the node. The input values flow to those layers, which ultimately calculate the final results.
Deep learning tunes the parameters in the hidden layers to achieve prediction results that are the same as the provided result. Many iterations are required for the machine-training process to achieve well-tuned parameters.
Every iteration includes the following actions:
- Run the prediction step using the input sample.
- Compare the prediction result against the provided result. The system will calculate how much difference between them, and this value is called loss.
- Modify the parameters in the hidden layers to minimize loss.
After the iterations are complete, you’ll have optimized parameters, and your results will have the highest possible precision.
Understanding Tensor and TensorFlow Prediction
For the training and prediction process, TensorFlow uses a data structure called Tensors as the input and output — hence why Tensor is a part of the name TensorFlow.
A Tensor is a multidimensional array that represents your input data and the machine-learning result.
The following definitions may help you understand what a tensor is, relative to what you already know:
- Scalar: Single value, for example:
1
,2
,3.3
- Vector: Multiple-axis value, examples:
(0, 0)
,(1, 2, 3)
- Tensor: Multiple-dimension value. Example is:
(((0, 0), (1, 0)), ((1,1), (2,2)))
In an image classification problem, the input tensor is an array that represents an image, similar to this:
[
// First line of the first image
[
// First Pixel of the first line
[0.0, 0.0, 1.0],
// Second Pixel of the first line
[0.0, 0.0, 1.0],
[1.0, 1.0, 0.0], ...
]
// Second line of the first image
...
...
]
To explain further:
- The first layer of the array represents every line of the image.
- The second layer of the array represents every pixel of the line.
- The last layer represents the color of the pixel, which is red, green, or blue.
If you resample the image to 200×200, the shape of the tensor is [200, 200, 3].
The output tensor is an array of the score for each label, for example:
[0.1, 0.8, 0.1, 0]
. In this case, each value corresponds to a label, for example, rose, tulip, sunflower and daisy.
Notice that in the example, the value for the tulip label is 0.8 — meaning the possibility that the image shows a tulip is 80%, the others are 10% and daisy 0%. The shape of the output here is [4]
.
The following diagram further illustrates the data flow:
Since TensorFlow uses tensors for the inputs and outputs, you need to do preprocessing so that TensorFlow understands the input data and postprocessing so that human users can understand the output data. You’ll install TensorFlow Lite in the next section to process the data.
Installing TensorFlow Lite in Flutter
To use TensorFlow in your Flutter app, you need to install the following packages:
- tflite_flutter: allows you to access the native TensorFlow Lite library. When you invoke the methods of tflite_flutter, it calls the corresponding method of the native TensorFlow Lite SDK.
- tflite_flutter_helper: enables you to manipulate TensorFlow inputs and outputs. For example, it converts image data to tensor structure. It reduces the effort required to create pre- and post-processing logic for your model.
Open pubspec.yaml and add them in the dependencies section:
tflite_flutter: ^0.9.0
tflite_flutter_helper: ^0.3.1
Then, run flutter pub get
to get packages.
Class 'TfliteFlutterHelperPlugin' is not abstract and does not implement abstract member public abstract fun onRequestPermissionsResult(p0: Int, p1: Array<(out) String!>, p2: IntArray)
it might be related to this issue. To work around it, replace the tflite_flutter_helper: ^0.3.1
dependency with the following git call:
tflite_flutter_helper:
git:
url: https://github.com/filofan1/tflite_flutter_helper.git
ref: 783f15e5a87126159147d8ea30b98eea9207ac70
tflite_flutter_helper:
git:
url: https://github.com/filofan1/tflite_flutter_helper.git
ref: 783f15e5a87126159147d8ea30b98eea9207ac70
Get packages again.
Then, if you are building for Android, run the installation script below on macOS/Linux:
./install.sh
If you’re on Windows, run install.bat
instead:
install.bat
However, to build for iOS, you need to download TensorFlowLiteC.framework, decompress it and place TensorFlowLiteC.framework in the .pub-cache folder for tflite_flutter. The folder location is /home/USER/.pub-cache/hosted/pub.dartlang.org/tflite_flutter-0.9.0/ios/, where USER is your username. If you’re not using version 0.9.0
, place it at the corresponding version.
You’re just adding dynamic Android and iOS libraries so that you can run TensorFlow Lite on your target platform.
Creating an Image Classifier
In machine learning, classification refers to predicting the class of an object out of a finite number of classes, given some input.
The Classifier
included in the starter project is a skeleton of the image classifier that you’ll create to predict the category of a given plant.
By the end of this section, the Classifier
will be responsible for these steps:
- Load labels and model
- Preprocess image
- Use the model
- Postprocess the TensorFlow output
- Select and build the category output
Your initialization code will load the labels and the model from your files. Then, it’ll build TensorFlow structures and prepare them to be used by a call to predict()
.
Your prediction action will include several parts. First, it’ll convert a Flutter image to a TensorFlow input tensor. Then, it’ll run the model and convert the output to the final selected category record that contains the label
and score
.
Classifier
instance. The last section of this tutorial, Using the Classifier, describes how it is implemented.Importing the Model to Flutter
There are two pieces of data that you’ll load into the program: the machine learning model – model_unquant.tflite and the classification labels — labels.txt, which you got from the Teachable Machine platform.
To begin, make sure to include the assets folder in pubspec.yaml:
assets:
- assets/
The assets
record is responsible for copying your resource files to the final application bundle.